Health Economics Modelling of Infectious Disease
a new mathematical model to evaluate utilities of different ways to prevent infectious disease such as COVID-19.
- Credit: First author
- Supervisor: Prof. Fan Zhang (XJTU)
- State: Submitted to attend XJTU ‘Tengfei Cup’ Technology Innovation Competition
- Time: May 2020 - May 2021
- Abstract: In this work, we employ mathematical modeling to analyze government funding allocation between clinical treatment and preventive medicine during epidemics. Findings suggest prioritizing clinical treatment for diseases like influenza with high prevalence and recovery rates, while prioritizing preventive medicine for low prevalence and recovery rate diseases like cancer. The comparison between disease prevalence and mortality rates should guide funding in cases of high prevalence but low recovery rate diseases–prioritizing clinical treatment for higher prevalence diseases like SARS, and preventive measures for higher mortality diseases like smallpox. Historical data and past epidemic case examples substantiate our conclusions.
- Key words: Clinical treatment, preventive medicine, government funding allocation, mathematical modeling, health Economics
- Download, Password: Arendelle-ftl
According to our model, the total loss of Society due to epidemics at time \(t\) is \(W(t)\)
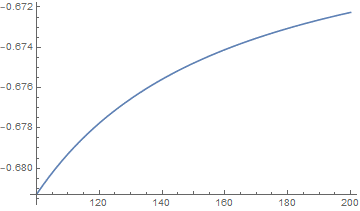
$$W(t), (100\le t\le 200)$$
Using Mathematica, we obtain
\[\lim_{t\to \infty}\left\lvert \frac{\frac{\partial W}{\partial \alpha}}{\frac{\partial W}{\partial \beta}}\right\rvert=\frac{\beta}{1-\alpha}\]in which \(\alpha\) is recovery rate and \(\beta\) is prevalence rate. So if \(\alpha+\beta >1\), we should prioritize clinical treatment, otherwise prioritize preventive measures.